Theory + AI Symposium
PI/1-100 - Theatre
Perimeter Institute for Theoretical Physics
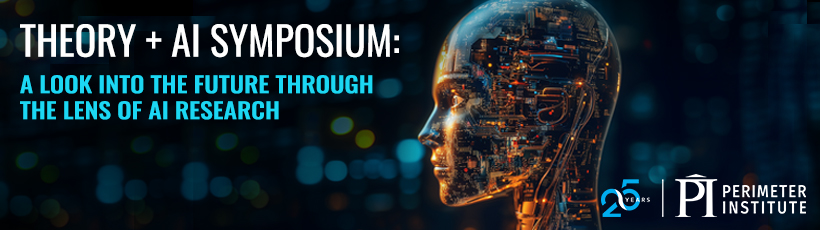
-
Frank Cappello (Argonne National Laboratory)
-
Yuri Chervonyi (Deep Mind)
-
Ioana Ciuca (Stanford University)
-
Deyan Ginev (LaTeXML)
- Geoffrey Hinton (University of Toronto)
- Shirley Ho (Polymathic & Simons Foundation)
-
Vicky Kalogera (Northwestern University)
-
Jared Kaplan* (Anthropic)
-
Peter Koepke (University of Bonn)
-
Roger Melko (University of Waterloo)
-
Moritz Munchmeyer (University of Wisconsin–Madison)
-
Axton Pitt (Litmaps)
-
Xiaoliang Qi (Stanford University)
-
Oleg Ruchayskiy (Niels Bohr Institute)
-
Gaurav Sahu (MILA)
-
Steinn Sigurdsson (arXiv)
-
Jesse Thaler (Massachusetts Institute of Technology)
-
Stephen Wolfram* (Wolfram Research)
-
Richard Zanibbi (Rochester Institute of Technology)
*virtual presentation
Scientific Organizers:
-
Matthew Johnson (Perimeter Institute)
-
Anindita Maiti (Perimeter Institute)
-
Sabrina Pasterski (Perimeter Institute)
Advisory Committee:
- Mykola Semenyakin (Perimeter Institute)